When I was eight years old, my parents decided that it was time to tell me Where Babies Came From. Cunningly, to avoid the embarrassment of a face-to-face conversation (it may have been the liberated 1960s, but I grew up in a vicarage), they left a sex education pamphlet lying around in the living room; as they knew I would, I pounced on it immediately and carried it off to my room for further attention. However, the further I got into the details, the more incredulous I became; obviously, this booklet had totally got the wrong end of the reproductive stick. Why on earth the author wanted to mislead innocent children I had no idea, but it was patently obvious that the acts described could not possibly be grounded in reality; how on earth was all this stuff supposed to happen when everyone knew that when you were in bed, you were always wearing pyjamas? For some days, I revelled in the intoxicating knowledge that I had discovered something that nobody else knew, until a conversation with my Dad put me straight.
That wonderful feeling that you alone out of all humanity have a vital piece of information that will revolutionise the world is the drug that drives not only misguided eight-year-olds, but scientific discovery. It took another 14 years before I got the same rush again, as a PhD student sequencing DNA. Every week in the lab, I stared at my autoradiographs stuffed full of sequencing data, knowing that nobody else had ever seen this information in the history of the world. Looking back, it was mundane stuff, but it felt wonderful. Like a flare lighting up the night sky, such moments continued to occasionally illuminate my career, and they made the day-to-day slog of lab life worthwhile.
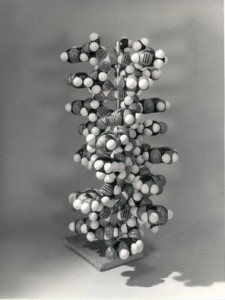
Linus Pauling’s proposed triple helix of DNA. Model built by Farooq Hussain. Image from http://tinyurl.com/nuwl6y3
Blue Skies and Benchspace is, of course, all about the firework moments in science, and many of the stories come from a golden era when the balance of excitement versus mundanity was less heavily skewed towards the latter than is the case today; life was a lot more fun, and a lot less cautious. The newness of the field led to an interesting philosophical difference between then and now: whilst people were not actively careless, and took tremendous pains to do things properly, the burden of proof was far less heavy than it is today. What mattered was rapid progress and discovery; if someone was wrong about something, as long as it was important, they’d be corrected quickly enough. Perhaps the most famous example of this is Linus Pauling’s 1953 paper suggesting that DNA was a triple helix, which was demolished only two months later by Watson and Crick.
Leaving a bit of leeway in one’s experiments in order to let oddities creep in was actively encouraged by Max Delbrück, one of the fathers of molecular biology, who trained his acolytes in the “Principle of Limited Sloppiness”. Delbrück’s “sloppy” did not mean careless, (although this too can lead to new discoveries if the perpetrator is careless but observant, pace Alexander Fleming) but rather, the word’s other definition, of sludgy, or muddy (interestingly, if Delbrück had expressed this idea in his native German, there would never have been any misunderstanding: careless in German is schlampig, whereas sludgy is schlammig). Leeway in experiments meant that unexpected results might ensue, as they did for the discovery of the DNA repair process enzymatic photoreactivation (for this great story, go to Chapter 4 and search on “sunlight”); if there hadn’t been uncontrolled differences in where the relevant experiments were done (sometimes in the dark, sometimes in sunlight), nobody would have realised that exposure to light was “curing” the damaged DNA.
Alongside limited sloppiness, it has to be said that many molecular biologists were somewhat contemptuous of what they saw as the ludicrous rigour with which other disciplines, particularly biochemists, conducted their experiments. In the early days at Caltech and Cold Spring Harbor, experimental controls were often done after the fact, and discussions about how to get things to work frequently included the enquiry “Have you tried adding pH to it?”, a faux-incompetent shorthand for “We’re all a bit too cool to be seen to be optimising conditions, but perhaps it’s worth a try”. Quite frequently, what with all the excitement, people did get stuff wrong. We all knew it; if you requested a reagent from another lab and didn’t bother to check that it was actually what it purported to be, you were a mug.
But was all of this a bad thing? When I started out in the 1980s, someone told me that one East Coast hotshot, when challenged on the dubious contents of a spectacular paper, is reputed to have said “We may have published it, but we never said it was right”. At the time, I dismissed this as arrogant tosh, which quite possibly it was; now, looking back, I’m starting to wonder whether getting things wrong might not be so awful, and indeed, should be an honourable occupational hazard of a discovery-led field.
I should emphasise at this point that of course, nobody ever wants to be wrong; many of the scientists who feature in Blue Skies and Bench Space mentioned that the time immediately after the first pulse-quickening realisation that they were looking at something entirely new was filled with dread that it was all some ghastly artefact. All went to great lengths to check their results, but even the best get it wrong, as David Page, one of the subjects of Chapter 8, found out when he misidentified the gene for male sex determination. His mistake was perfectly pardonable; given the data he had, and the previous literature on the topic, there was really no way he could have known he’d made an error. Similarly, p53, which features in Chapter 3, was thought for years to cause cancer, rather than suppress it, thanks to a misconception shared by almost an entire field.
So, nobody wants to be wrong, but everyone knows that for reasons beyond your control (or even within your control), you might be. This tacit acknowledgement is the reason that all scientific statements are hedged about with qualifiers. Read a good paper, and you’ll see phrases such as “the data support the conclusion that X may be true”, “we present evidence suggesting that Y interacts with Z”, “these results imply that A is the case”. Hedging in this way is a necessary corollary of the urgency of getting exciting data out there, establishing a bridgehead into the scientific unknown that you and your colleagues can then consolidate, or otherwise. If everyone waited till they were 100% sure of a result, practically nothing would ever be published, and scientific progress would slow to a crawl.
There is obviously a big caveat here. Although one might accept that error in some types of discovery-led research is permissible, and indeed, necessary, for rapid progress to occur, when it comes to clinically relevant research with a direct impact on human health, mistakes should be kept to an absolute minimum as lives are at stake. And this is where contemporary research is falling down. There are too many examples of dodgy cell lines, and inappropriate model systems, where results have been squeezed out by questionable statistical analyses. Mistakes in analysing and/or overinterpreting the mountain of data being generated from the large-scale sequencing of DNA, RNA, proteins and metabolites are also common, given that the methodology for transforming the raw numbers into something coherent is far beyond the grasp of many users.
Biology is of necessity becoming far more quantitative, and to be anything other than a monumentally expensive pile of junk, the numbers have to be right. For example, if it appears from analysis of DNA sequences from multiple tumour samples that a particular gene is mutated in a substantial number of patients, the analysis had better be right, or lives, time and money may be wasted. Data concerning potential drug targets must be rigorously validated, and all-to-frequently, attempts to replicate results have been unsuccessful. Two recent articles from scientists working at the drug companies Amgen and Bayer Healthcare found that only 11% and 25% respectively of the academic preclinical research they examined was correct. These shockingly low percentages undoubtedly contribute to failed drug trials; sometimes the drug target is simply irrelevant to the disease process. Of course, pharmaceutical companies are not whiter than white in this respect; one would have thought they would check a bit more carefully before committing billions to developing a drug, and some are also guilty of distorting clinical trial data by withholding negative results.
There is a theory, most eloquently propounded by John Ioannidis of the University of Ioannina in Epirus, Greece, that suggests that the high levels of error are a consequence of the current culture in medical research. In essence, Ioannidis proposes that a publication process which rewards extreme, spectacular results by exposure in high impact factor journals and enhanced job and funding prospects, is riding for a fall, as there will be a tendency to neglect the less exciting data which may give a truer picture. A proper discussion of his ideas, together with his proposals as to how this state can be remedied can be found here. I fervently hope that one day, science will work out how to achieve a proper balance between risk-taking and caution, but until then, it’s worth remembering that results can be ranked as follows: correct, and outstandingly interesting; wrong, but still outstandingly interesting; solid and correct; a bit sloppy, but still correct, and, lagging a long way behind all those three categories: shoddy, dull and empty of content. Sadly, too many papers in the latter category are still slipping through the net.
NB. Obviously (I hope), what I’ve written above does not deal with the additional problem of faking data, which can never, ever be considered acceptable.
Discussion
No comments yet.